KEY POINTS-
- Information processing may occur at multiple levels, from microtubules to brain-wide cell assemblies.
- Artificial "neural networks" may be similar to genuine brain processes or they may be simply metaphors.
- Cell assemblies can form and dissolve in fractions of seconds, altering connections at the speed of thought.
- Integrated Information Theory is an interesing but controversial idea with many supporters and detractors.
Does consciousness arise exclusively from complex interactions in brain tissue (materialism)? Or does consciousness originate in some more fundamental level of reality (dualism)? Many nuanced philosophical versions of these positions have surfaced in recent years. The new science of consciousness remains consistent with established physical laws, while fully embracing the knowledge barriers and "hidden reality" imposed by modern physics.
Computer science contributes to this debate through developments in artificial intelligence (AI). A substantial blurring of distinctions between materialism and dualism may be in the works. One source of shifting viewpoints stems from a deeper appreciation of the role of information in both the physical and biological sciences. Information and consciousness appear to be close relatives, some even view them as marriage partners. Prominent scientists and philosophers may claim that “consciousness is just brain-wide information sharing.” But just what is this enigmatic entity “information,” a label often embraced with more enthusiasm than critical scrutiny?
Scientists naturally search for ways to identify, and hopefully even measure, some well-defined “intrinsic information content” of brains. One of today’s leading theories of consciousness was discussed briefly in my post of December 13, 2021, Consciousness and Integrated Information Theory (IIT), a controversial idea with plenty of supporters and detractors. One major issue for any information-based theory is that the meaning of “brain information” is generally subjective and context-dependent.
Information processing may involve multiple levels of organization, ranging from protein molecules, including the tiny microtubules, to cellular scales, to brain-wide cell assemblies to global fields of synaptic action. These nested structures can form and dissolve in fractions of seconds, changing their organizations and mutual alliances at the speed of thought. Brain structures are often loosely labeled as “neural networks,” but their relationships to AI networks might be anything from genuine physical analogs to loose metaphorical similarities. My purpose in this new series of posts is to examine possible relations between information and consciousness, starting at the most basic level so that the discussion is easily available to any interested reader.
Let’s first visit the “binary world” of information. Binary numbers are physically convenient. Bones placed on the ground, beads sliding on sticks, and punched holes in paper tapes have long been used to store and manipulate binary information. Punch cards were the standard means of inputting binary data to early computers. When punched properly, each card location either has a hole or no hole. A famous example of binary misuse involved the “hanging chads” of the 2000 U.S. presidential election. The world of binary information has taken on deep scientific significance—in the second law of thermodynamics, quantum mechanics, biological systems, and more.
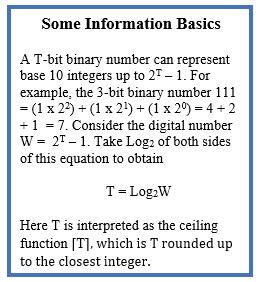
Imagine sand buckets placed in a straight line on a beach. Empty buckets are designated 0 and sand-filled buckets are designated 1; fractional fills are not allowed. I adopt the symbol T (after computer scientist Alan Turing) to indicate the number of buckets in the line. As shown in my first figure, a T-bit binary number can represent base-10 integers up to the maximum number W = 2T – 1. Solving this equation for T yields the minimum number of bits (buckets or binary digits) needed to store the number W: T = Log2W. The right side is read, “the base 2 logarithm of W”. This equation has no fixed scientific meaning; it is simply the rule for transforming any base-10 number W into a binary number T, analogous to a simple mathematical rule like A x B = B x A. The binary transformation rule adopts the ceiling function of computer science, the provision that non-integer values of T are to be rounded up to the nearest integer. Thus, for example, ceiling function (6.2) = 7 bits or buckets; no partly filled buckets or hanging chads are allowed.
State Information
A system’s state is the collection of features that describe its condition, at a fixed time or, more likely, over a specified time average. This simple definition masks an extensive assortment of subtle issues that must be faced head-on in the quest to better understand consciousness. State information may be defined simply by the number of possible states of a system, let’s say water, which can be found in the states of solid, liquid, or gas. Economies, forests, and ant colonies can exist in different states. Brains can occupy many mental states—awake, dreaming, Alzheimer’s disease, planning a bank robbery, or thinking great thoughts. Neuroscience seeks relationships between mental states and measurable physical states. Such relationships are labeled the neural correlates of consciousness.
Let’s begin our discussion of states with simple die systems. If a single cubical die is placed or tossed on a surface, the six possible states of the system are 1 through 6. A polyhedron die is a three-dimensional object with W flat surfaces. If, for example, W = 128, each system state can be unequivocally labeled by a single positive integer in the range 1 through 128. This state description is independent of the causes or probabilities of occurrence of each state, which might be the result of a simple die toss, deliberate placement by an intelligent being, or some hidden process. We only require that the system be found in one of the 128 discrete states, independent of the cause or means of observation. The size of state information is defined simply as W, the number of distinct states that the system can be in. To be more precise, we may call W the size of the die's macro-state to distinguish it from the micro-states, which are determined by the locations and velocities of the elementary particles forming the die. State size can also be expressed by T, the number of bits corresponding to W.
Shannon Entropy
State information is often confused with Shannon information (or entropy) developed in modern communication theory. According to Shannon information, a message consists of a sequence of M symbols selected from an alphabet consisting of N symbols. For example, the 7-bit computer code ASCII can contain up to N = 128 symbols. Consider the message, “The Bird is cruel!”, which probably means nothing much to the reader unless he or she has read science fiction by Robert Heinlein. The message consists of M = 18 symbols, including letters, spaces, and an exclamation point. In the common practice of sending messages, meaning is supplied by both the message content and prior knowledge of the receiver. In contrast, the central goal of communication theory is to provide quantitative measures of the information content of messages, independent of meaning.
A message’s Shannon information is defined in terms of the probability of occurrence of each symbol produced by the sending system (whether human or machine). For example, the letter E is more likely to occur than other letters in English text. The information transmitted by each symbol involves a degree of receiver surprise; the letter Z conveys more information than the letter E and so forth. Shannon information is, by definition, additive. Each additional symbol received adds more information, but the additional information varies in size, depending on symbol probabilities in the chosen alphabet. Shannon entropy H is the average number of bits of information per transmitted symbol. If an entire alphabet were to contain only a single symbol, H would be zero. On the other hand, if all symbols were to have an equal probability of occurrence, Shannon entropy takes on its maximum value: H = Log2M. More generally, the Shannon entropy H of any collection of symbols, including but not limited to alphabets, lies in the range between zero and Log2M, depending on the probability distribution of the alphabet. H may or may not have any relationship to the intrinsic properties of the sender.
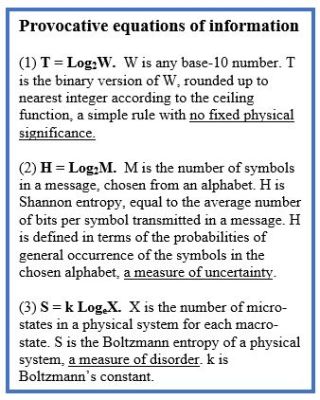
Three major kinds of information are defined in figure 2: T (Turing buckets), H (Shannon entropy), and S (Boltzmann entropy). The three equations are nearly identical but express very different physical processes. The discovery of entropy in the mid 1800s was followed by the development of statistical mechanics in the late 1800s and Shannon’s communication theory in 1949. Many seem to have fallen for the fallacy that T, H, and S are the same creature because they satisfy (essentially) the same equation. In this regard, note that mechanical and electrical systems are often described by identical differential equations, providing the basis for analog computers.
Widespread confusion about information and entropy also originates from their inherently abstract nature, mixed interpretations of the symbols H and S, and the births and on-going maturation of many new informational siblings. For example, Boltzmann's sharp distinction between the macro and micro-states in classical statistical mechanics is not fully adequate to describe complex systems.
Brains other complex systems operate at multiple intermediate scales, leading to multi-scale entropy measures. The confusion continues to this day, especially with the advent of AI, quantum computing with quantum bits (qubits), quantum superposition of states, black hole entropy, cellular information processing, and much more. In future posts, I will discuss other kinds of information that appear to be related to consciousness.
Several efforts to find better-defined measures of intrinsic brain information have tentatively adopted Shannon entropy by interpreting the symbols of the Shannon message (M) as measured brain states (W), adopting Shannon entropy (H) as an approximation of the brain’s intrinsic information. One obvious problem with this approach is that the contents of a message may tell us almost nothing about the internal states of the sender. If the sender is a very simple system, like our 128-face die, its message may provide much of the die’s intrinsic macro-scale information. However, in brains as well as other complex systems, the relationships between the system messages (often obtained from experimental data) and the intrinsic information of the system is tenuous at best. I will look into these issues in more depth in my next post.